MBL MEL IV: Eight resources
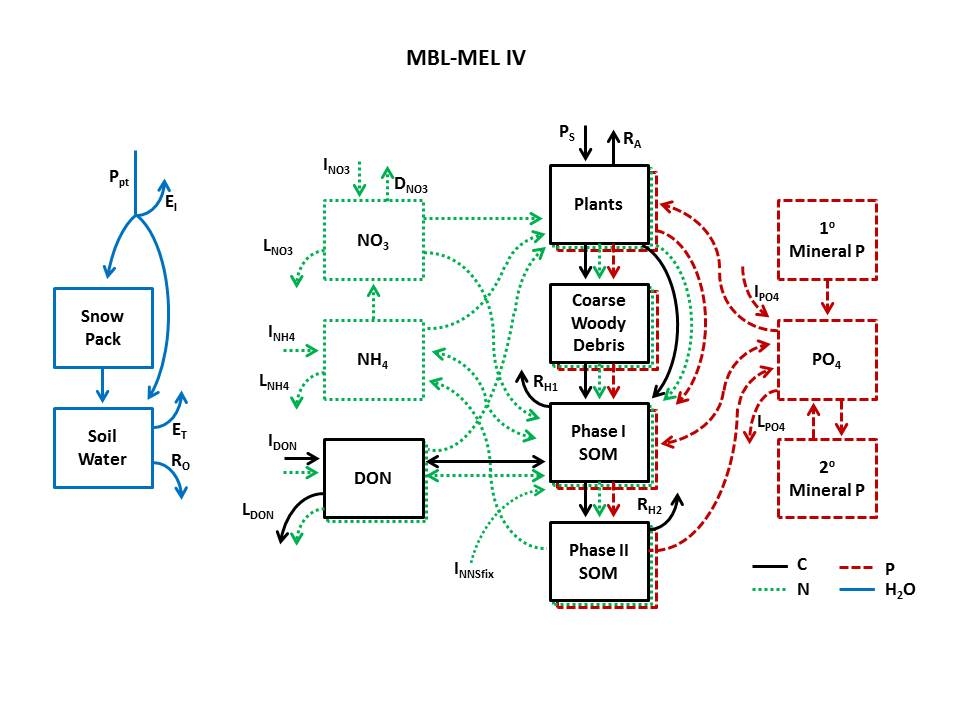
MBL MEL IV broadens the application of the resource optimization hypothesis to interactions among more than two resources, including non-element resources (e.g., light and water), and among substitutable resources (e.g., NH4 or NO3 as sources of N). The approach is general enough that the model can now be extended to any number of element and non-element resources and to any number of substitutable resources.
MBL MEL IV couples ecosystem carbon (C), nitrogen (N), phosphorus (P), and water cycles and runs on a daily time step. We use an aggregate representation of vegetation, partitioned allometrically into woody and active biomass. The algorithm that redistributes uptake effort allocates the active biomass to leaves or fine roots based on the relative limitation by canopy vs. soil resources (Rastetter et al. 2001). Photosynthesis is co-limited by CO2, light, and water. Nutrient uptake follows Michaelis-Menten kinetics with the substrate concentration at the root set by a balance between uptake and nutrient diffusion through the soil and maximum uptake set by the expended effort.
We use uptake effort to represent the aggregate of all plant assets (such as biomass, enzymes, carbohydrate) that can be deployed toward the acquisition of resources. The abundance of these assets increases in proportion to the active biomass in the vegetation and the dynamic allocation algorithm calculates the fraction of these uptake assets (Vi) allocated toward acquiring each resource (i). Uptake is proportional to both Vi and the total amount of active biomass.
The allocation of effort is based on the current requirements for, and the current uptake rates of, the resources. The requirement for each resource equals its consumption in metabolism plus its loss in tissue turnover times a factor that compensates for deviations of the resource concentrations in biomass from an optimum concentration. We calculate this optimum concentration from the allometric distribution of biomass among tissues and parameters defining the optimum concentrations in the tissues (parameters qLN, qWN, qRN, qLP, qWP, and qRP, where q is the biomass optimum of N or P (subscripts) in leaves, woody tissues, and roots (subscripts L, W, and R, respectively)). The dynamic resource optimization scheme allocates uptake effort among resources based on a function Ωi that increases monotonically with the ratio of resource requirement to resource uptake:
where Vi is the fraction of uptake effort allocated toward resource i, a is an acclimation rate parameter (day-1), and Φ is a variable that is calculated to ensure that the dVi/dt sum to zero and thereby keeps the sum of the Vi equal to 1. This allocation scheme drives the system toward a state where the ratios of uptake to requirement are equal for all resources and are in that sense equally limiting (i.e., Ωi=1/Φ for all i; Bloom et al. 1985).
For the substitutable resources, we calculate the requirement for each based on the total requirement and the marginal yield from allocated effort for each, including the effort needed to acquire resources consumed in uptake and assimilation of each resource.
where yi is the marginal yield for resource i, Ui is the uptake of resource i, ϕ is the amount of resource j consumed in the uptake and assimilation of resource i, and Vj is the total effort allocated toward the acquisition of resource j. To optimize resource acquisition, the allocation of effort is toward the resource with the highest marginal yield.
We partition detritus into Phase I and Phase II soil organic matter (Melillo et al. 1989) and coarse woody debris. Coarse woody debris serves as a storage for woody litter that is slowly converted to Phase I soil organic matter (SOM) before it begins to decompose (Hyvönn and Ågren 2001). Phase I SOM represents the young, more active organic matter that implicitly includes microbial biomass, both mineralizes and immobilizes nutrients, and has a relatively high respiration rate. Phase I material also converts to Phase II material at a rate that at steady state is equivalent to ~20% of the coarse plus fine litter flux (i.e., 20% of the initial litter is eventually converted to Phase II material; Melillo et al. 1989). Phase II SOM does not immobilize nutrients, but continues to mineralize nutrients and respire C at a slow rate. Nitrogen enters the ecosystem via deposition of inorganic and organic N. Phosphorus enters as PO43– via deposition or the weathering of primary and secondary P minerals. Nitrogen and phosphorus are mineralized from both Phase I and Phase II material into NH4+ and PO43–. We partition the NH4+, PO43–, and dissolved organic N into adsorbed and dissolved fractions using a Langmuir isotherms (Weatherley and Miladinovic 2004); rates of leaching and uptake by plants and microbes are calculated based on the dissolved fraction. We assume no NO3- sorption by soils (Nodvin et al. 1986, Kaiser and Zech 1996) and that all the NO3- is therefore dissolved in soil water and is available for uptake by plants and microbes, leaching, and denitrification.
We partition precipitation into intercepted water, snow, and rain. We calculate a maximum potential intercepted volume based on the leaf area plus the surface area of the aboveground woody biomass. We assume that all precipitation up to this maximum volume is intercepted and evaporates. Precipitation in excess of this maximum value is added either to the snow pack or to the soil water, depending on air temperature (Brubaker et al. 1996). We calculate snowmelt based on net radiation to the snowpack and air temperature (Brubaker et al. 1996). Runoff from the soil water is proportional to the volume of water in excess of field capacity. Soil water potential is calculated based on equations from Clapp and Hornberger (1978). We calculate soil water uptake and transpiration by plants as proportional to the soil water potential above the wilting potential and proportional to an index of the daily vapor pressure deficit; the proportionality is set by the allocation of effort toward water uptake in the resource optimization scheme.
Applications
Rastetter, E.B., R.D. Yanai, R.Q. Thomas, M.A. Vadeboncoeur, T.J. Fahey, M.C. Fisk, B.L. Kwiatkowski, and S.P. Hamburg. 2013. Recovery from disturbance requires resynchronization of ecosystem nutrient cycles. Ecological Applications. 23:621-642. doi:10.1890%2F12-0751.1
Download the model description, model executable, parameter and driver files for all figures.
Pearce, AR, EB Rastetter, WB Bowden, MC Mack, Y Jiang, and BL Kwiatkowski. 2015. Recovery of arctic tundra from thermal erosion disturbance is constrained by nutrient accumulation: a modeling analysis. Ecological Applications 25:1271-1289.
Jiang, Y, EB Rastetter, AV Rocha, AR Pearce, BL Kwiatkowski, GR Shaver. 2015. Modeling Carbon-Nutrient interactions during the early recovery of tundra after fire. Ecological Applications 25:1640-1652.
Jiang, Y., A.V. Rocha, J.A. O’Donnell, J. A. Drysdale, E. B. Rastetter, G. R. Shaver, and Q. Zhuang. In press. Contrasting soil thermal responses to fire in Alaskan tundra and boreal forest. JGR-Earth Surface
Jiang, Y, AV Rocha, EB Rastetter, GR Shaver, U Mishra, Q Zhuang, BL Kwiatkowski. In press. C-N-P interactions control climate driven changes in regional patterns of C storage on the North Slope of Alaska. Landscape Ecol. DOI 10.1007/s10980-15-0266-5
Jiang, Y., E.B. Rastetter, G.R. Shaver, A.V. Rocha, Q. Zhuang, and B.L. Kwiatkowski. in review, Long-term changes in tundra carbon balance following wildfire, climate change and potential nutrient addition, a modeling analysis. Ecological Applications.
A study investigating the mechanisms that control long-term response of tussock tundra to fire and to increases in air temperature, CO2, nitrogen deposition and phosphorus weathering. The simulations indicate that the recovery of nutrients lost during wildfire is difficult under a warming climate because warming increases nutrient cycles and subsequently leaching within the ecosystem.
Download the model description, model executable, parameter and driver files.
Output files are available from the Arctic LTER database (hyperlink will be active once the files are available).
Citations
Bloom, A. J., F. S. Chapin, III, and H. A. Mooney. 1985. Resource limitation in plants—an economic analogy. Annual Review of Systematics and Ecology 16:363–392.
Brubaker, K., A. Rango, and W. Kustas. 1996. Incorporating radiation inputs into the snowmelt runoff model. Hydrological Processes 10:1329–1343.
Clapp, R. B., and G. M. Hornberger. 1978. Empirical equations for soil hydraulic properties. Water Resources Research 14:601–604.
Hyvönen, R., and G. I. Ågren. 2001. Decomposer invasion rate, decomposer growth rate, and substrate chemical quality: how they influence soil organic matter turnover. Canadian Journal of Forest Research 31:1594–1601.Kaiser, K., and W. Zech. 1996. Nitrate, sulfate, and biphosphate retention in acid forest soils affected by natural dissolved organic carbon. Journal of Environmental Quality 25:1325–1331.
Melillo, J. M., J. D. Aber, A. E. Linkins, A. Ricca, B. Fry, and K. J. Nadelhoffer. 1989. Carbon and nitrogen dynamics along the decay continuum: Plant litter to soil organic matter. Plant and Soil 115:189–198.
Nodvin, S. C., C. T. Driscoll, and G. E. Likens. 1986. Simple partitioning of anions and dissolved organic carbon in a forest soil. Soil Science 142:27–35.
Rastetter, E. B., P. M. Vitousek, C. Field, G. R. Shaver, D. Herbert, G. I. Ågren. 2001. Resource Optimization and Symbiotic N Fixation. Ecosystems 4:369-388. doi:http://dx.doi.org/10.1007%2Fs10021-001-0018-z
Weatherley, L. R., and N. D. Miladinovic. 2004. Comparison of the ion exchange uptake of ammonium ion onto New Zealand clinoptilolite and mordenite. Water Research 38:4305–4312.
This material is based upon work supported by the National Science Foundation under grants #OPP-9318529, OPP-9732281, DEB-9509613, DEB 0716067 (OPUS), DEB-0108960, ARC-0806329, EF-1065587, ARC-0856853, and DEB-0949420 and the Environmental Protection Agency under grants RFQ-RT-00-00107 and QT-RT-00-001667. Any opinions, findings, conclusions, or recommendations expressed in the material are those of the author(s) and do not necessarily reflect the views of the National Science Foundation, the Environmental Protection Agency or the Marine Biological Laboratory.